class: center, middle, inverse, title-slide .title[ # Introduction to tidymodels workflow ] .author[ ### Chun Su ] .institute[ ### R-ladies Philly ] .date[ ### 2022-07-14 ] --- class: center, middle ## Keep in touch [<svg viewBox="0 0 512 512" style="height:1em;position:relative;display:inline-block;top:.1em;" xmlns="http://www.w3.org/2000/svg"> <path d="M464 64H48C21.49 64 0 85.49 0 112v288c0 26.51 21.49 48 48 48h416c26.51 0 48-21.49 48-48V112c0-26.51-21.49-48-48-48zm0 48v40.805c-22.422 18.259-58.168 46.651-134.587 106.49-16.841 13.247-50.201 45.072-73.413 44.701-23.208.375-56.579-31.459-73.413-44.701C106.18 199.465 70.425 171.067 48 152.805V112h416zM48 400V214.398c22.914 18.251 55.409 43.862 104.938 82.646 21.857 17.205 60.134 55.186 103.062 54.955 42.717.231 80.509-37.199 103.053-54.947 49.528-38.783 82.032-64.401 104.947-82.653V400H48z"></path></svg> sckinta@gmail.com](mailto:sckinta@gmail.com) [<svg viewBox="0 0 496 512" style="height:1em;position:relative;display:inline-block;top:.1em;" xmlns="http://www.w3.org/2000/svg"> <path d="M165.9 397.4c0 2-2.3 3.6-5.2 3.6-3.3.3-5.6-1.3-5.6-3.6 0-2 2.3-3.6 5.2-3.6 3-.3 5.6 1.3 5.6 3.6zm-31.1-4.5c-.7 2 1.3 4.3 4.3 4.9 2.6 1 5.6 0 6.2-2s-1.3-4.3-4.3-5.2c-2.6-.7-5.5.3-6.2 2.3zm44.2-1.7c-2.9.7-4.9 2.6-4.6 4.9.3 2 2.9 3.3 5.9 2.6 2.9-.7 4.9-2.6 4.6-4.6-.3-1.9-3-3.2-5.9-2.9zM244.8 8C106.1 8 0 113.3 0 252c0 110.9 69.8 205.8 169.5 239.2 12.8 2.3 17.3-5.6 17.3-12.1 0-6.2-.3-40.4-.3-61.4 0 0-70 15-84.7-29.8 0 0-11.4-29.1-27.8-36.6 0 0-22.9-15.7 1.6-15.4 0 0 24.9 2 38.6 25.8 21.9 38.6 58.6 27.5 72.9 20.9 2.3-16 8.8-27.1 16-33.7-55.9-6.2-112.3-14.3-112.3-110.5 0-27.5 7.6-41.3 23.6-58.9-2.6-6.5-11.1-33.3 2.6-67.9 20.9-6.5 69 27 69 27 20-5.6 41.5-8.5 62.8-8.5s42.8 2.9 62.8 8.5c0 0 48.1-33.6 69-27 13.7 34.7 5.2 61.4 2.6 67.9 16 17.7 25.8 31.5 25.8 58.9 0 96.5-58.9 104.2-114.8 110.5 9.2 7.9 17 22.9 17 46.4 0 33.7-.3 75.4-.3 83.6 0 6.5 4.6 14.4 17.3 12.1C428.2 457.8 496 362.9 496 252 496 113.3 383.5 8 244.8 8zM97.2 352.9c-1.3 1-1 3.3.7 5.2 1.6 1.6 3.9 2.3 5.2 1 1.3-1 1-3.3-.7-5.2-1.6-1.6-3.9-2.3-5.2-1zm-10.8-8.1c-.7 1.3.3 2.9 2.3 3.9 1.6 1 3.6.7 4.3-.7.7-1.3-.3-2.9-2.3-3.9-2-.6-3.6-.3-4.3.7zm32.4 35.6c-1.6 1.3-1 4.3 1.3 6.2 2.3 2.3 5.2 2.6 6.5 1 1.3-1.3.7-4.3-1.3-6.2-2.2-2.3-5.2-2.6-6.5-1zm-11.4-14.7c-1.6 1-1.6 3.6 0 5.9 1.6 2.3 4.3 3.3 5.6 2.3 1.6-1.3 1.6-3.9 0-6.2-1.4-2.3-4-3.3-5.6-2z"></path></svg> @sckinta](http://github.com/sckinta) [<svg viewBox="0 0 448 512" style="height:1em;position:relative;display:inline-block;top:.1em;" xmlns="http://www.w3.org/2000/svg"> <path d="M313.6 304c-28.7 0-42.5 16-89.6 16-47.1 0-60.8-16-89.6-16C60.2 304 0 364.2 0 438.4V464c0 26.5 21.5 48 48 48h352c26.5 0 48-21.5 48-48v-25.6c0-74.2-60.2-134.4-134.4-134.4zM400 464H48v-25.6c0-47.6 38.8-86.4 86.4-86.4 14.6 0 38.3 16 89.6 16 51.7 0 74.9-16 89.6-16 47.6 0 86.4 38.8 86.4 86.4V464zM224 288c79.5 0 144-64.5 144-144S303.5 0 224 0 80 64.5 80 144s64.5 144 144 144zm0-240c52.9 0 96 43.1 96 96s-43.1 96-96 96-96-43.1-96-96 43.1-96 96-96z"></path></svg> learniningwithsckinta.netlify.app](https://learniningwithsckinta.netlify.app) [<svg viewBox="0 0 512 512" style="height:1em;position:relative;display:inline-block;top:.1em;" xmlns="http://www.w3.org/2000/svg"> <path d="M458.4 64.3C400.6 15.7 311.3 23 256 79.3 200.7 23 111.4 15.6 53.6 64.3-21.6 127.6-10.6 230.8 43 285.5l175.4 178.7c10 10.2 23.4 15.9 37.6 15.9 14.3 0 27.6-5.6 37.6-15.8L469 285.6c53.5-54.7 64.7-157.9-10.6-221.3zm-23.6 187.5L259.4 430.5c-2.4 2.4-4.4 2.4-6.8 0L77.2 251.8c-36.5-37.2-43.9-107.6 7.3-150.7 38.9-32.7 98.9-27.8 136.5 10.5l35 35.7 35-35.7c37.8-38.5 97.8-43.2 136.5-10.6 51.1 43.1 43.5 113.9 7.3 150.8z"></path></svg> R-Ladies Philly](https://www.rladiesphilly.org/) --- class: center, middle ### The [**tidymodels**](https://www.tidymodels.org/) is _a collection of packages_ for modeling and machine learning using <span style="color:red">tidyverse</span> principles. --- ## ML steps in <span style="color:red">**tidymodels**</span> workflow .pull-left[ - resampling : [`rsample`](https://rsample.tidymodels.org/) - preprocess : [`recipes`](https://recipes.tidymodels.org/) - modeling : [`parsnip`](https://parsnip.tidymodels.org/) - tune hyperparameters: [`tune`](https://tune.tidymodels.org/) - evaluation : [`yardstick`](https://yardstick.tidymodels.org/) ] <br> <br> <br> .pull-right[ <img src="https://workflows.tidymodels.org/logo.png" style="width: 30%" /> ] <img src="https://cdn.quantargo.com/assets/courses/course-r-machine-learning-tidymodels/01-basics/images/tidymodels_process.svg" style="width: 100%" /> - connect everything together: [`workflows`](https://workflows.tidymodels.org/) and [`workflowsets`](https://workflowsets.tidymodels.org/) --- ## Make data budget - Initial split to training (75%) and testing (25%) data ```r library(tidyverse) library(tidymodels) data("wa_churn") *set.seed(123) data_split <- initial_split( wa_churn, prop = 3/4, * strata = churn ) data_train <- training(data_split) data_test <- testing(data_split) ``` -- <br> .center[`set.seed`: make split reproducible] -- <br> .center[`strata`: enforcing stratified sampling] -- <br> .center[`training` vs. `testing`] ??? by default, it split 75% This is more common with classification problems where the response variable may be severely imbalanced (e.g., 90% of observations with response “Yes” and 10% with response “No”). However, we can also apply stratified sampling to regression problems for data sets that have a small sample size and where the response variable deviates strongly from normality (i.e., positively skewed like Sale_Price). With a continuous response variable, stratified sampling will segment into quantiles and randomly sample from each. Consequently, this will help ensure a balanced representation of the response distribution in both the training and test sets. we do not use the test set to assess model performance during the training phase. So how do we assess the generalization performance of the model? --- ## Budget training data with resampling <br> <br> -- .center[.large[**what is resampling?**]] -- > An alternative approach by allowing us to repeatedly fit a model of interest to parts of the training data and test its performance on other parts. <br> <br> -- .center[ .large[**why do we perform resampling?**] ] -- > To check the variability of model and assess model performance during the training phase. ??? fit more accurate models, model selection and parameter tuning. we do not use the test set to assess model performance during the training phase. So how do we assess the generalization performance of the model --- ## resample methods in <span style="color:red">**rsample**</span> - k-fold cross-validation (CV) <img src="https://bradleyboehmke.github.io/HOML/images/cv.png" style="width: 100%" /> .footnote[https://bradleyboehmke.github.io/] -- ```r set.seed(123) data_folds <- vfold_cv(data_train, strata = churn, v = 10) ``` --- ## resample methods in <span style="color:red">**rsample**</span> - bootstrap <img src="https://bradleyboehmke.github.io/HOML/images/bootstrap-scheme.png" style="width: 100%" /> .footnote[https://bradleyboehmke.github.io/] -- ```r set.seed(123) data_boots <- bootstraps(data_train, times = 25, strata = Y) ``` ??? the same size as the original data set from which it was constructed. --- ## Feature engineering and preprocessing .center[.large[>The features you use influence more than everything else the result. No algorithm alone, to my knowledge, can supplement the information gain given by correct feature engineering. — *Luca Massaron*]] <img src="https://imageio.forbes.com/blogs-images/gilpress/files/2016/03/Time-1200x511.jpg" style="width: 100%" /> -- ```r data_rec <- recipe(churn ~ ., data = data_train) ``` ??? feature engineering can make or break an algorithm’s predictive ability and deserves --- ## Feature engineering with <span style="color:red">**recipes**</span> - dealing with missing data (imputation) ```r data_rec %>% step_impute_knn(all_predictors(), neighbors = 6) ``` -- - feature filtering: remove variance "zero" features ```r data_rec %>% step_zv(all_predictors()) ``` -- - numeric feature transformation and normalization ```r data_rec %>% step_log() %>% step_normalize(all_numeric_predictors()) ``` -- - decode qualitative predictors ```r data_rec %>% step_dummy(all_nominal_predictors()) ``` --- ## Feature engineering with <span style="color:red">**recipes**</span> - create new features - add interactive term - define roles - dimension reduction - ... .footnote[https://recipes.tidymodels.org/reference/] -- ```r data_rec <- data_rec %>% step_impute_knn(total_charges, neighbors = 6) %>% step_mutate_at(c("female", "senior_citizen", "partner", "dependents", "phone_service", "paperless_billing"), fn=function(x){as.factor(ifelse(x==0, "No", "Yes"))}) %>% step_normalize(tenure, monthly_charges, total_charges) %>% step_dummy(all_nominal_predictors()) data_rec %>% prep() %>% juice() # view preprocessed data ``` <br> -- .center[**Data pre-process must be done on the training set only in order to avoid [data leakage](https://www.kaggle.com/code/alexisbcook/data-leakage/tutorial)**] ??? Filter out zero or near-zero variance features. Perform imputation if required. Normalize to resolve numeric feature skewness. Standardize (center and scale) numeric features. Perform dimension reduction (e.g., PCA) on numeric features. One-hot or dummy encode categorical features. https://machinelearningmastery.com/data-leakage-machine-learning/ --- ## Specify models with <span style="color:red">**parsnip**</span> ```r lm_spec <- linear_reg() %>% # define a model set_engine("lm") %>% # select an engine (require packages installed) set_mode("regression") # select a mode ``` .footnote[https://parsnip.tidymodels.org/reference/] -- Two modes of modeling: - regression - classification -- *Some models can be used in more than one mode. It is a good practice to specify each model with `set_mode`* <br> -- <br> *The same model can be fit with different engines where hyperparameters name can be different accordingly* --- ## Hyperparameters vs. Parameters <img src="https://www.hitechnectar.com/wp-content/uploads/2020/04/Hyperparameter-vs.-Parameter-Differences-Tabular-Diagram.jpg" style="width: 100%" /> .small[https://www.hitechnectar.com/blogs/hyperparameter-vs-parameter] --- ## Tune hyperparameters with <span style="color:red">tune</span> and <span style="color:red">dial</span> - Identify which hyperparameters to tune in model specification ```r rf_spec <- rand_forest( * mtry = tune(), * min_n = tune() ) %>% set_engine( "ranger", importance = "permutation" # for feature vip ) %>% set_mode("classification") ``` .footnote[https://tune.tidymodels.org/reference/] -- - Create a grid of values for hyperparameters ```r rf_grid <- grid_regular( * mtry(range = c(1L, 10L)), # mtry need upbound specified * min_n(), levels = 3 ) ``` --- ## Tune hyperparameters in <span style="color:red">workflows</span> `workflows` stitch all elements together ```r rf_wf <- workflow() %>% # create a workflow * add_model(rf_spec) %>% # add model specification * add_recipe(data_rec) # add data recipes set.seed(234) # for random forest fit rf_res <- rf_wf %>% * tune_grid( * resamples = data_folds, # use resample cv folds to tune * grid = rf_grid, # hyperparameters grid control = control_grid(save_workflow = T) # save workflow ) rf_res %>% head(5) ``` ``` ## # A tibble: 5 × 4 ## splits id .metrics .notes ## <list> <chr> <list> <list> ## 1 <split [4752/529]> Fold01 <tibble [18 × 6]> <tibble [0 × 1]> ## 2 <split [4753/528]> Fold02 <tibble [18 × 6]> <tibble [0 × 1]> ## 3 <split [4753/528]> Fold03 <tibble [18 × 6]> <tibble [0 × 1]> ## 4 <split [4753/528]> Fold04 <tibble [18 × 6]> <tibble [0 × 1]> ## 5 <split [4753/528]> Fold05 <tibble [18 × 6]> <tibble [0 × 1]> ``` -- .center[.bold[Question: how many times the model was fitted in our case?]] --- ## Evaluate hyperparameters ```r rf_res %>% collect_metrics() %>% head(10) ``` ``` ## # A tibble: 10 × 8 ## mtry min_n .metric .estimator mean n std_err .config ## <int> <int> <chr> <chr> <dbl> <int> <dbl> <chr> ## 1 1 2 accuracy binary 0.738 10 0.000973 Preprocesso… ## 2 1 2 roc_auc binary 0.827 10 0.00896 Preprocesso… ## 3 5 2 accuracy binary 0.795 10 0.00424 Preprocesso… ## 4 5 2 roc_auc binary 0.836 10 0.00680 Preprocesso… ## 5 10 2 accuracy binary 0.788 10 0.00457 Preprocesso… ## 6 10 2 roc_auc binary 0.827 10 0.00674 Preprocesso… ## 7 1 21 accuracy binary 0.741 10 0.00131 Preprocesso… ## 8 1 21 roc_auc binary 0.828 10 0.00866 Preprocesso… ## 9 5 21 accuracy binary 0.800 10 0.00370 Preprocesso… ## 10 5 21 roc_auc binary 0.844 10 0.00646 Preprocesso… ``` --- ## Evaluate hyperparameters 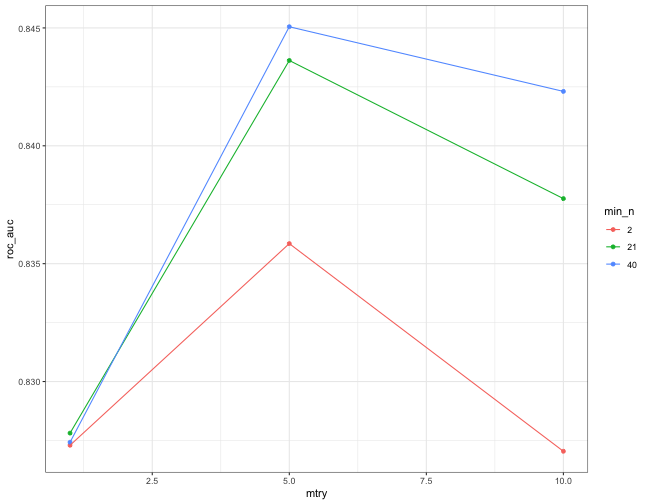<!-- --> --- ## finalize workflow and evaluate model with <span style="color:red">yardstick</span> - finalize workflow ```r best_rf <- rf_res %>% select_best(metric = "roc_auc") final_wf <- rf_wf %>% finalize_workflow(best_rf) ``` -- - last fit to test data ```r set.seed(123) # for random forest fit final_fit <- final_wf %>% last_fit(data_split) ``` -- - evaluate final fit on test data using `yardstick` ```r final_fit %>% collect_predictions() %>% roc_auc(churn, .pred_Yes) ``` ``` ## # A tibble: 1 × 3 ## .metric .estimator .estimate ## <chr> <chr> <dbl> ## 1 roc_auc binary 0.844 ``` --- ## ROC curve on prediction of test data .pull-left[ ```r final_fit %>% collect_predictions() %>% roc_curve(churn, .pred_Yes) %>% autoplot() ``` ] .pull-right[ <img src="intro2tmwf_xaringan_files/figure-html/unnamed-chunk-22-1.png" style="display: block; margin: auto;" /> ] --- ## Predict on new data using trained workflow - Returns the trained workflow object ```r final_wf_trained <- final_fit %>% * extract_workflow() ``` -- .center[required the control option `save_workflow = TRUE` was used in `tune_grid`] .center[The trained workflow object can be saved for future prediction] <br> -- - predict on new data ```r final_wf_trained %>% * augment(new_data = wa_churn[1:5,]) %>% select(churn, contains(".pred_")) ``` .center[`augment` vs. `predict`] --- ## Importance of variables .pull-left[ ```r library(vip) final_wf_trained %>% extract_fit_parsnip() %>% vip() + theme_bw() ``` ] .pull-right[ 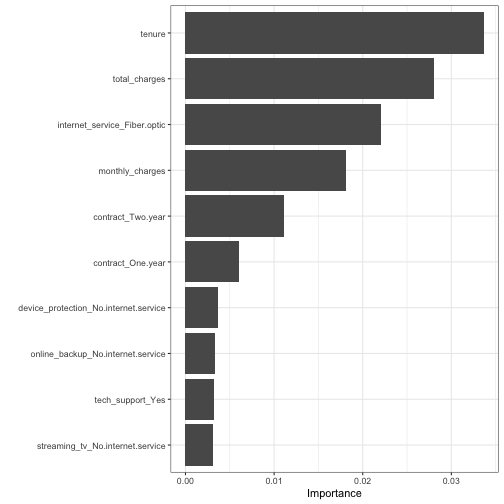<!-- --> ] --- ## Reference - [Tidymodels Get Started](https://www.tidymodels.org/start/) - [Hands-On Machine Learning with R](https://bradleyboehmke.github.io/HOML/) by Bradley Boehmke & Brandon Greenwell - [An Introduction to Machine Learning with R](https://lgatto.github.io/IntroMachineLearningWithR/index.html) by Laurent Gatto - [Tidy Modeling with R](https://www.tmwr.org/) by Max Kuhn & Julia Silge - [Hyperparameter vs. Parameter: Difference Between The Two](https://www.hitechnectar.com/blogs/hyperparameter-vs-parameter) by Kelsey Taylor - [Data Leakage in Machine Learning](https://machinelearningmastery.com/data-leakage-machine-learning/) by Jason Brownlee